
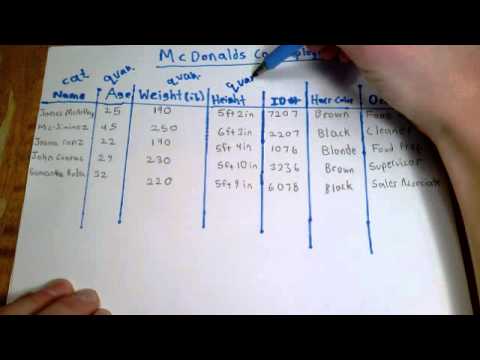
The use of significant variables such as the month, cooling degree days (CDD), heating degree days (HDD), and GDP explained 94.7% of the variation in electricity demand. formed regression models and employed convolutional neural networks (CNNs) to forecast long-term electricity demand predictions for the state of Florida. There are some interesting items for different forecast horizons. The literature on modeling and load prediction using linear regression techniques is rich. Short-term load forecasting (STLF), which is investigated most often, usually aims to predict the load from one day to up to one week ahead, and very-short-term load forecasting (VSTLF) is used for forecasts with the time horizons of less than 24 h. Medium-term load forecasting (MTLF) uses hourly loads to predict the weekly peak load for both power and system operations planning. Long-term load forecasting (LTLF) is used to predict the annual peak of the power system in order to optimize future investments in construction and launching of new units required to meet nationwide electricity demand over time periods of up to 20 years. In general, load forecasting has been investigated by utilities and electricity suppliers. The choice of the appropriate technique and method for load forecasting depends largely on the forecast horizon. The scope of electricity consumption forecasting techniques and methodologies is quite large. The terms load, demand, power, and energy consumption are often used alternately in different articles dealing with energy modeling and forecasting. In addition, we conclude that the model can also detect days with unusual energy consumption. We obtained a mean relative uncertainty of 1.72% in the learning data set, and 1.69% and 1.82% in the two testing data sets, respectively. We show that for about 84% of the data points, the relative error of the model is below 2.5%, and for all the data points the error does not exceed 7.5%. The applied model shows that four hours are sufficient to describe energy consumption during the remainder of a given day. This model is self-configurable and very fast. The number of data points required to describe a whole day’s consumption depends on the demanded precision, which is up to the user. The proposed technique provides a way to accurately model day-to-day energy consumption using just a few selected variables. This paper presents a new approach concerning hourly energy consumption using a multivariate linear regression model. On the other hand, the research on load modeling and forecasting methodologies is quite limited. At present, papers concerning energy consumption and forecasting are predominantly dedicated to various known techniques and their combinations.
